We use cookies to understand how you use our site and to improve the overall user experience. This includes personalizing content and advertising. Read our Privacy Policy
Machine Learning
CD Genomics is good at using various cutting-edge analysis algorithms and machine learning methods to integrate and analyze various basic research and clinical data. With its efficient and accurate characteristics, machine learning helps biomedical research and promotes the development of the industry.
Introduction
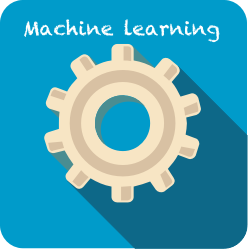
The definition of machine learning is "a computer program obtains an evaluation P by performing some tasks T, thereby obtaining experience E. If the program can obtain a better experience E through tasks T and evaluation P, then we learn this program and perform machine learning." The effectiveness of machine learning often depends on the amount of data and the quality of the data. Large amounts of high-quality data can produce better results. With the development of the medical industry, a large amount of clinical data and scientific research data have been generated. Big data and machine learning are based on better decision-making, optimized and innovative features, which greatly improve the efficiency of scientific research and clinical trials of doctors or researchers. Through the collection of clinical or scientific research data recorded by R&D departments, doctors and clinics, patients, nurses, etc., the integrated analysis is carried out using machine learning methods. It can be used to improve or solve some problems in the infrastructure and treatment level of healthcare. The application of machine learning in biology is mainly divided into supervised learning and unsupervised learning.
Machine Learning Methods
- Supervised learning is to learn a function from a given training data set. When new data arrives, the result can be predicted based on this function. In layman's terms, the way to determine whether it is supervised learning is to determine whether the researcher wants an answer. A very important category in supervised learning is the classification problem. In the classification problem, the variable that you want to predict is discrete, not continuous. For example, data on the size of the tumor, the age of the patient, and whether the tumor is benign or malignant.
- Compared with supervised learning, unsupervised learning has no artificially labeled results in the training set. The purpose of machine learning is to find certain rules from these data. An important part of unsupervised learning is the clustering algorithm. For example, if you want to distinguish different groups in a mixed biological or medical sample, it is to use unsupervised learning.
Applications of Machine Learning in Biomedical Research
- In basic research:
- In Clinical Research:
- Disease identification/diagnosis: The identification of diseases and the diagnosis of pain are the cutting-edge applications of machine learning research in medicine.
- Personalized treatment/behavior modification: For example, the use of supervised learning algorithms allows doctors to choose from a limited set of diagnoses, or evaluate the risks faced by the patient based on the patient's symptoms and genetic information.
- Drug discovery/production: From the initial screening of drug compounds to the prediction of success rate based on biological factors, the use of machine learning in preliminary (early) drug discovery has multiple application potentials.
- Clinical trial research: such as identifying candidates for clinical trials, collecting data in a wider range including, for example, social media and doctor follow-up.
- Radiology and radiotherapy: Use machine learning algorithms to detect the difference between healthy and cancerous tissues.
- Intelligent electronic health records: machine learning-based technologies such as the use of support vector machines to classify medical documents (such as sorting patient queries based on emails) and optical character recognition (to convert cursive or other deformed handwriting into digital characters.
Fig1. Application of machine learning in basic research.
What We Offer
CD Genomics provides high-quality one-stop data analysis services according to customers' data analysis needs. The machine learning analysis services we provide cover a variety of different types of data, such as related research and development data, pathological data, test reports, and data recorded by nursing staff. Utilizing ultra-large-scale computing resources and various cutting-edge analysis algorithms, we select the appropriate analysis algorithm model for customers according to the data analysis requirements, and finally obtain reliable and useful analysis results. For our data analysis services, if you have any questions, please feel free to contact our professional technical support. We are always ready to provide you with satisfactory services.
Our workflow
References
- Badillo S, et al. An Introduction to Machine Learning[J]. Clin Pharmacol Ther. 2020 Apr;107(4):871-885.
- Alonso-B A, et al, Big-Data Analysis, Cluster Analysis, and Machine-Learning Approaches[J]. Adv Exp Med Biol. 2018; 1065:607-626.
* For research use only. Not for use in clinical diagnosis or treatment of humans or animals.
Online Inquiry
Please submit a detailed description of your project. Our industry-leading scientists will review the information provided as soon as possible. You can also send emails directly to info@cd-genomics.com for inquiries.